Laiye discusses language models
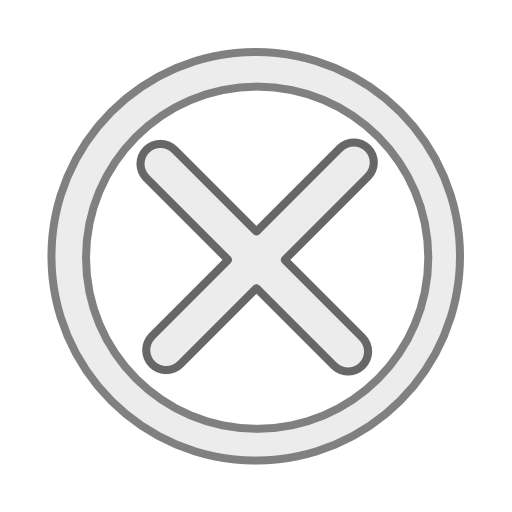

Pierre Pakey, head of product innovation at Laiye Technology (Beijing) Co Ltd, shared his thoughts about how large language models, a new path in artificial intelligence, can mimic human minds in some ways during the latest Vision China.
Previously, the most common way to train AI was to give it plenty of examples, a process called supervised training.
With the new approach of descriptive training, AIs are trained in the same way humans would be, by describing the task that requires completion in natural language, Pakey said.
Taking the example of intelligent document processing, where the goal is to extract key information such as issue dates, supplier addresses and vendor names from an invoice, he said that the common way of training involves feeding the AI thousands of invoices.
But as invoices vary, one of the issues is physically pinpointing the positions of each piece of information on the documents in order to train the language model. This process is both slow and prone to errors, Pakey said.
"With descriptive training, people just describe what they want in plain language. So it's extremely simple and it completely changes the time necessary to actually launch a new AI and train on a new task," he said.
With descriptive training, users must ask themselves what the best question is, and what is the best way of asking the model to perform the desired task. This process is called prompt engineering.
"The first time we launched a large language model in production, we had very disappointing accuracy, meaning that our metrics were telling us this was a bad model. When we dove deeply to understand why our accuracy was poor, we noticed that the model was still doing better than the human laborers it was ranked against," he said.
The large language model needed to handle hundreds and even thousands of different examples, and in this situation, it did better than human workers at labeling.
As with any AI model, its accuracy did not exceed 95 percent, and Pakey said that if users needed further improvements, it was only a question of aligning expectations and of being very clear about what information they wanted to extract.
Large language models still have limitations. They need to be given time to produce a good answer, and when prompting different models for an answer, it's important to bear in mind the trade off between complexity and accuracy, Pakey said.
"But most importantly, they are able to learn new tasks almost instantly, and that makes this one of the most exciting things that we have seen in AI in a long time."