AI reshapes race for digital sovereignty
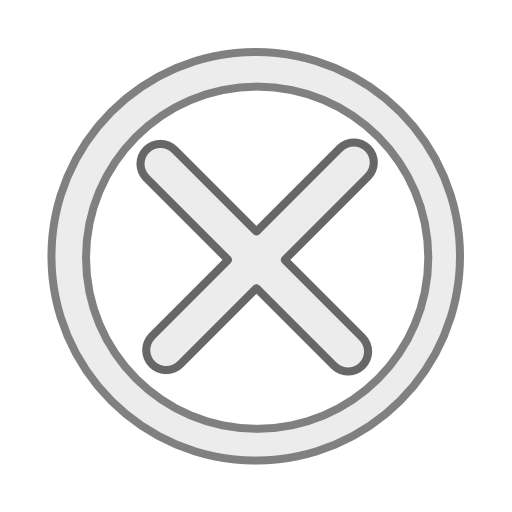
Fundamental challenge
The fundamental challenge for improving AI remains compute and efficiency.
The current foundation models have already hit a scaling wall, where exponentially more compute is required to achieve some improvements in performance.
Empirical research suggests that doubling compute typically results in only a 10 to 20-percent reduction in loss functions — illustrating the rapidly diminishing returns of brute-force scaling. This scaling frontier makes innovations in model architecture and algorithms indispensable.
Conversely, DeepSeek or the focus on compute efficiency will not reduce the need for powerful hardware, nor should it justify the weaponization of chips as "chokehold technology".
The next generation of AI models — capable of humanlike reasoning, acting as autonomous agents and tackling complex applications — will require significantly more computational power.
Nvidia's Huang estimates that computational demand for advanced AI systems has already increased by a factor of 100 within a year.
Global AI computing demand is projected to reach 864 ZFLOPS (a unit for measuring the speed of a computer system) by 2030, a 4000-fold increase over 2000 levels, underscoring the need for continued investment in high-performance computing infrastructure.